Improvement of Neural Networks Applied to Photoacoustic Signals of Semiconductors with Added Noise
Само за регистроване кориснике
2021
Аутори
Đorđević, Кatarina Lj.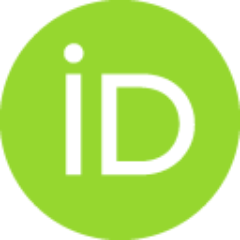
Galović, Slobodanka
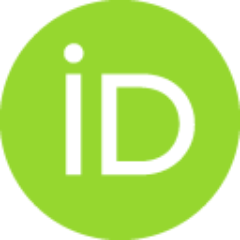
Jordović-Pavlović, Miroslava I.
Ćojbašić, Žarko М.
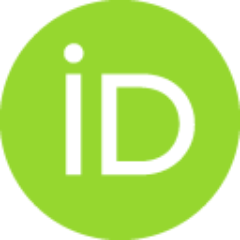
Markushev, Dragan D.
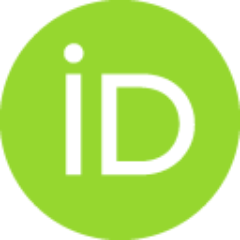
Чланак у часопису (Објављена верзија)
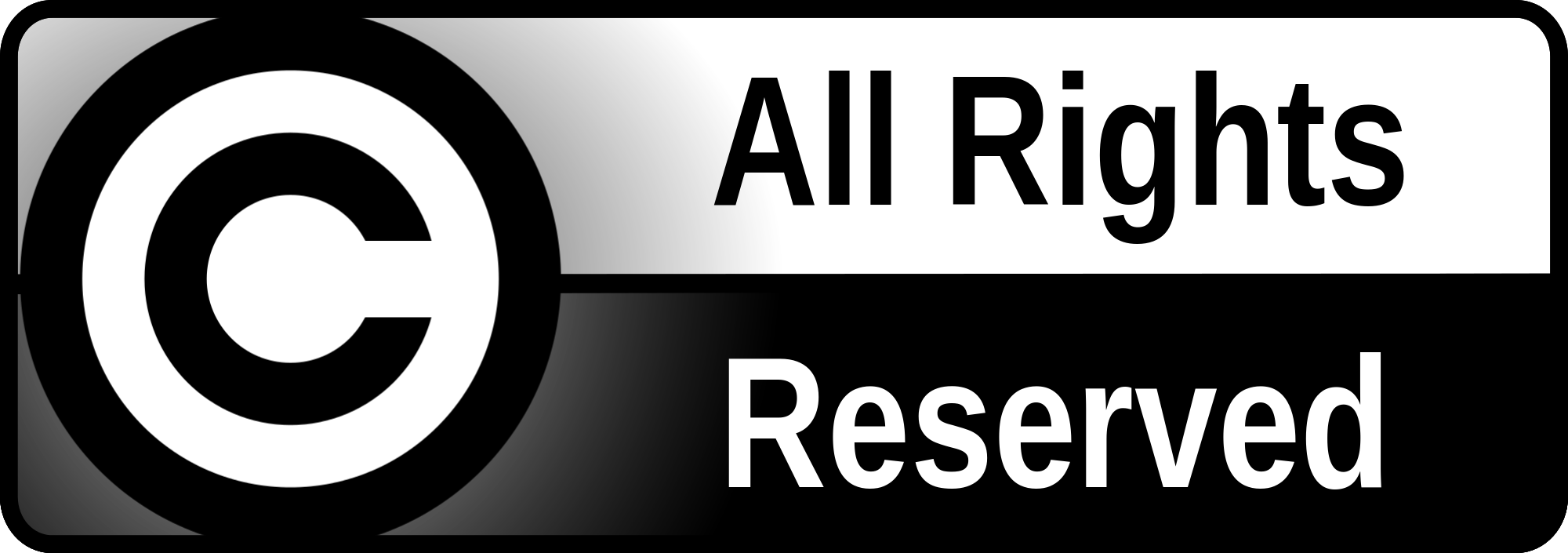
Метаподаци
Приказ свих података о документуАпстракт
This paper provides an overview of the characteristics of different neural networks trained on the same theoretical database of n-type silicon photoacoustic signals. By adding different levels of random Gaussian noise to the training input signals, two important goals were achieved. First, the optimal level of noise was found which significantly shortens the training networks with minimal loss of accuracy of its predictions. Second, the termination criteria of networks training were activated to avoid overtraining, i.e., networks generalization was performed. A networks efficiency analysis was performed on both theoretical and experimental photoacoustic signals, resulting in a selection of one neural network that is optimal to the performance requirements of the real experiment. It is indicated that the application of such trained networks is more reliable on thicker semiconductors, whose thickness is greater than the value of the carrier diffusion length in the investigated sample. © ...2020, Springer Nature B.V.
Кључне речи:
Artificial neural networks / Gaussian random noise / Inverse problem / n-type silicon / Photoacoustic / Photothermal / Semiconductors / Thermal diffusion / Thermal expansionИзвор:
Silicon, 2021, 13, 9, 2959-2969Финансирање / пројекти:
- Атомски сударни процеси и фотоакустичка спектрометрија молекула и чврстих тела (RS-MESTD-Basic Research (BR or ON)-171016)
- Функционални, функционализовани и усавршени нано материјали (RS-MESTD-Integrated and Interdisciplinary Research (IIR or III)-45005)
DOI: 10.1007/s12633-020-00606-y
ISSN: 1876-990X
WoS: 000560303400003
Scopus: 2-s2.0-85089463678
Колекције
Институција/група
VinčaTY - JOUR AU - Đorđević, Кatarina Lj. AU - Galović, Slobodanka AU - Jordović-Pavlović, Miroslava I. AU - Ćojbašić, Žarko М. AU - Markushev, Dragan D. PY - 2021 UR - https://vinar.vin.bg.ac.rs/handle/123456789/9581 AB - This paper provides an overview of the characteristics of different neural networks trained on the same theoretical database of n-type silicon photoacoustic signals. By adding different levels of random Gaussian noise to the training input signals, two important goals were achieved. First, the optimal level of noise was found which significantly shortens the training networks with minimal loss of accuracy of its predictions. Second, the termination criteria of networks training were activated to avoid overtraining, i.e., networks generalization was performed. A networks efficiency analysis was performed on both theoretical and experimental photoacoustic signals, resulting in a selection of one neural network that is optimal to the performance requirements of the real experiment. It is indicated that the application of such trained networks is more reliable on thicker semiconductors, whose thickness is greater than the value of the carrier diffusion length in the investigated sample. © 2020, Springer Nature B.V. T2 - Silicon T1 - Improvement of Neural Networks Applied to Photoacoustic Signals of Semiconductors with Added Noise VL - 13 IS - 9 SP - 2959 EP - 2969 DO - 10.1007/s12633-020-00606-y ER -
@article{ author = "Đorđević, Кatarina Lj. and Galović, Slobodanka and Jordović-Pavlović, Miroslava I. and Ćojbašić, Žarko М. and Markushev, Dragan D.", year = "2021", abstract = "This paper provides an overview of the characteristics of different neural networks trained on the same theoretical database of n-type silicon photoacoustic signals. By adding different levels of random Gaussian noise to the training input signals, two important goals were achieved. First, the optimal level of noise was found which significantly shortens the training networks with minimal loss of accuracy of its predictions. Second, the termination criteria of networks training were activated to avoid overtraining, i.e., networks generalization was performed. A networks efficiency analysis was performed on both theoretical and experimental photoacoustic signals, resulting in a selection of one neural network that is optimal to the performance requirements of the real experiment. It is indicated that the application of such trained networks is more reliable on thicker semiconductors, whose thickness is greater than the value of the carrier diffusion length in the investigated sample. © 2020, Springer Nature B.V.", journal = "Silicon", title = "Improvement of Neural Networks Applied to Photoacoustic Signals of Semiconductors with Added Noise", volume = "13", number = "9", pages = "2959-2969", doi = "10.1007/s12633-020-00606-y" }
Đorđević, К. Lj., Galović, S., Jordović-Pavlović, M. I., Ćojbašić, Ž. М.,& Markushev, D. D.. (2021). Improvement of Neural Networks Applied to Photoacoustic Signals of Semiconductors with Added Noise. in Silicon, 13(9), 2959-2969. https://doi.org/10.1007/s12633-020-00606-y
Đorđević КL, Galović S, Jordović-Pavlović MI, Ćojbašić ŽМ, Markushev DD. Improvement of Neural Networks Applied to Photoacoustic Signals of Semiconductors with Added Noise. in Silicon. 2021;13(9):2959-2969. doi:10.1007/s12633-020-00606-y .
Đorđević, Кatarina Lj., Galović, Slobodanka, Jordović-Pavlović, Miroslava I., Ćojbašić, Žarko М., Markushev, Dragan D., "Improvement of Neural Networks Applied to Photoacoustic Signals of Semiconductors with Added Noise" in Silicon, 13, no. 9 (2021):2959-2969, https://doi.org/10.1007/s12633-020-00606-y . .